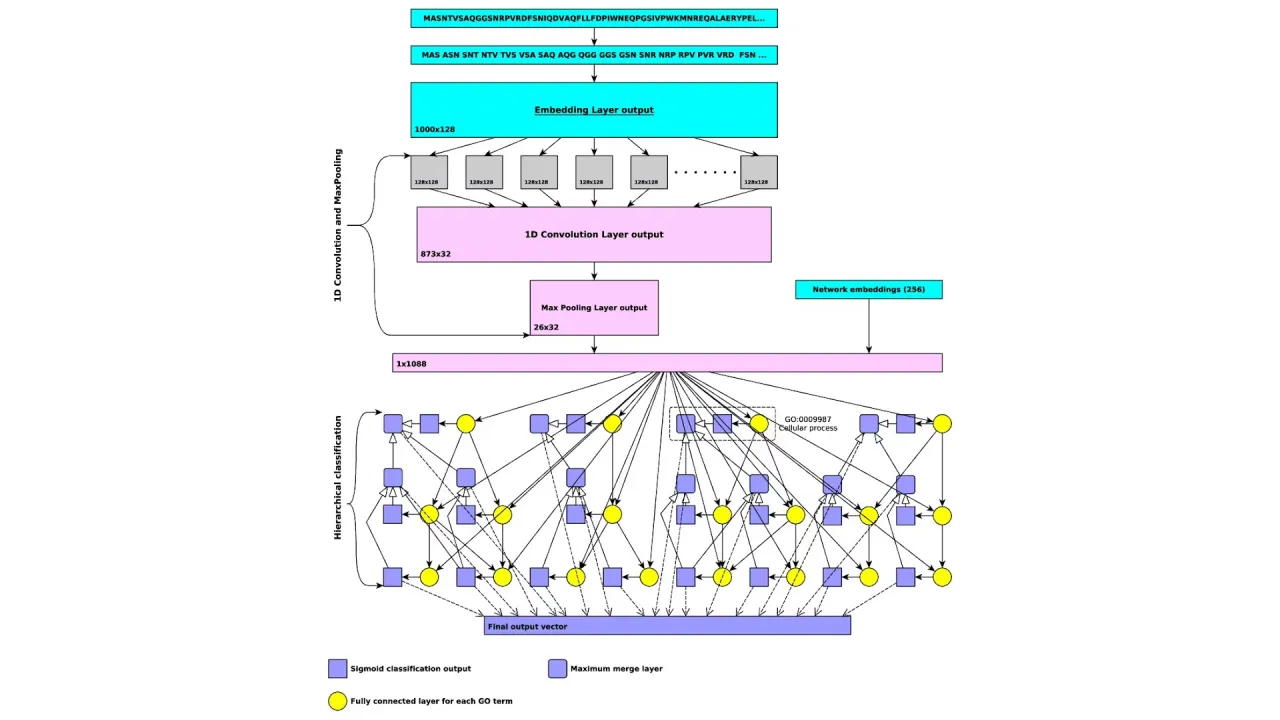
Prediction of functions and phenotypes
Overview
Details
The amount of available protein sequences is rapidly increasing, mainly as a consequence of the development and application of high throughput sequencing technologies in the life sciences. It is a key question in the life sciences to identify the functions of proteins, and furthermore to identify the phenotypes that may be associated with a loss (or gain) of function in these proteins. Protein functions are generally determined experimentally, and it is clear that experimental determination of protein functions will not scale to the current – and rapidly increasing – amount of available protein sequences (over 300 million). Furthermore, identifying phenotypes resulting from loss of function is even more challenging as the phenotype is modified by whole organism interactions and environmental variables. It is clear that accurate computational prediction of protein functions and loss of function phenotypes would be of significant value both to academic research and to the biotechnology industry.
In our project, we will develop and expand novel methods for predicting protein functions and their loss of function phenotypes. We will utilize deep neural network algorithm and combine them with symbolic inference into neural-symbolic algorithms. Our work will significantly extend our previously developed method for predicting protein functions called DeepGO through methodological advances in machine learning, incorporation of broader data types that may be predictive of functions, and improved systems for neural-symbolic integration.
We will apply our methods to the prediction of protein function for metagenomic samples of the Red Sea in order to evaluate the potential for the discovery of novel proteins of industrial value. We will also apply our methods to the prediction of loss of function phenotypes in human genetics and incorporate the results in a variant prioritization tool that can be applied to diagnose patients with Mendelian disorders.
The methods we will develop are generic and can be applied to other domains in which similar types of structured and unstructured information exist.
Collaborators
- Nicholas Dimonachos, Aberystwyth University